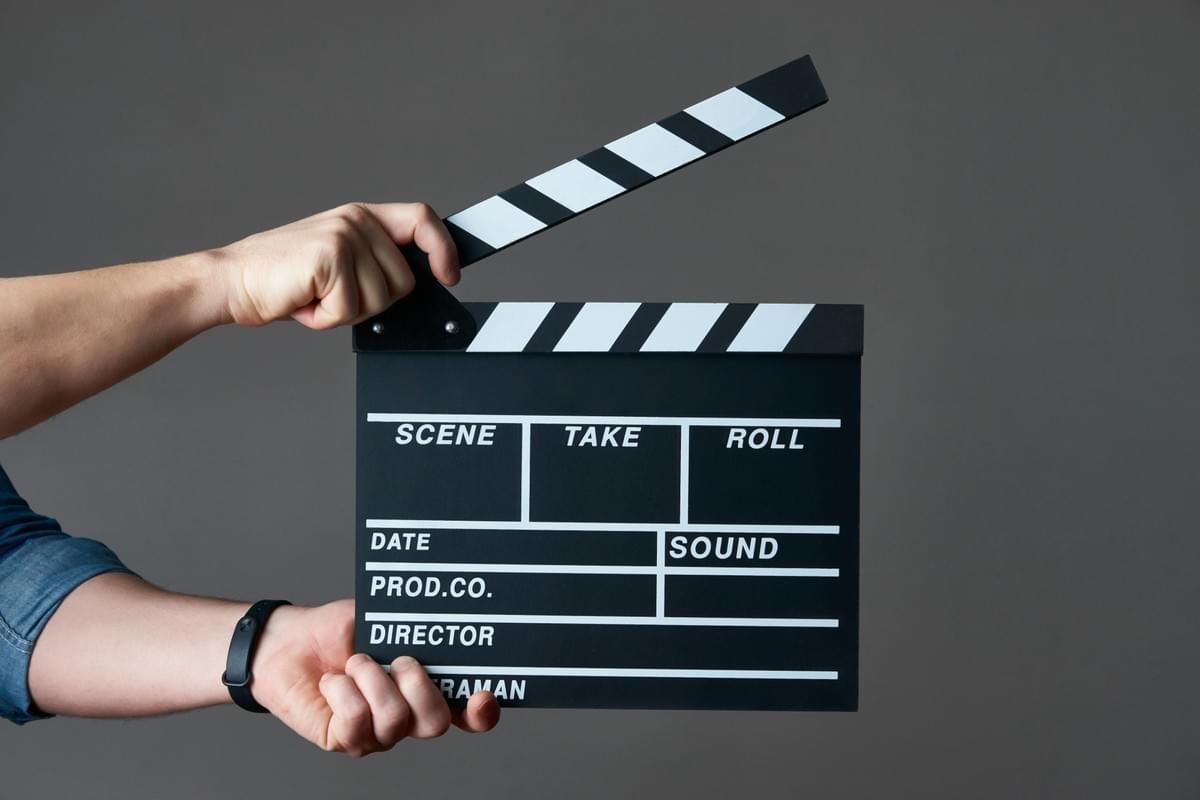
One of the most convenient and enjoyable ways to discover new movies is by browsing recommendations on movie websites. Unlike the traditional methods of finding a good film in a search engine, websites like Movie of the Night take the work out of recommending films. Aside from offering detailed reviews of the movies, they also allow you to filter results based on the rating on IMDb, Metacritic, and streaming services. Additionally, you can search by genre, year of release, and primary language. After you've narrowed down your options, you'll see a list of recommended movies based on your preference. Continue reading this article to get more insight on how to get an ideal movie website.
For example, consider two users A and B, who both watched the same movie. The recommendation system will return similar results for both users. In this scenario, user A can find a movie recommended to him by user B if the two users share some preferences. In this example, user A's recommendation would be the same as that of user B. In contrast, user B's recommendation would be a negative correlation, indicating that the two users have nothing in common. Therefore, a user-to-user collaborative filtering system is best suited for mainstream movies. Click this link to get the best movie website you can rely on: http://thebobapp.com/.
In practice, recommender systems are useful for recommending movies based on similarity of users. They help to classify items based on the similarity of users. In addition, movies recommended by one user can be recommended to another user. However, it is essential to note that the recommendations are not guaranteed to be relevant to another user. A recommendation system that makes use of the Movielens dataset can help you decide which movies are worth watching - and which ones are not.
The popularity of a particular film can help determine whether it is worth watching. When users give a high rating to a movie, the algorithm will consider this factor. If two users rate it highly, the recommendation system will recommend that movie to the other. If they haven't seen it, the recommendation system will not recommend it to the other user. Instead, it will recommend a movie that is more similar to the user's choice.
The same algorithm can be used to classify similar users. For example, a user's ratings can be used to determine which movies are related to his or her own preferences. A good example of this is a recommendation system that recommends a movie based on the preferences of the users. Then the system can search a database to find similar movies. By looking for movies of similar characteristics, the recommendation system will be able to recommend a certain type of film to other users.
Generally, users who share the same preferences will be recommended similar movies. This way, they will not have to waste time searching for a similar movie. In fact, the recommendation system will suggest a similar movie to the user. When it comes to movies, the same rule holds true for taste. Moreover, the same user may have different tastes and likes. Some people may prefer niche movies, while others might prefer classic movies. They may be interested in a particular genre. Get more insight about this topic here: https://en.wikipedia.org/wiki/Film.